AI Test Myths vs. Reality: Best Tools for Automation
Artificial intelligence (AI) is transforming nearly every industry, but confusion around AI testing continues to create roadblocks. Many assume an AI test is just another automation tool — but that myth can cost businesses real innovation. In truth, understanding how an AI test works—and how to use it strategically—can unlock immense potential, especially for any AI development company or business looking to stay competitive.
In this guide, we’ll explore what AI testing really means, debunk common misconceptions, and introduce the best tools for automating your testing workflows. Whether you’re new to artificial AI applications or looking to scale AI for business, this breakdown is designed to help you move forward with confidence.
Quick Navigation:
- ⚙️ What Is an AI Test?
- 🔍 AI Testing vs. Traditional Testing
- 🚀 Why AI Testing Tools Matter
- 📌 Best Use Cases
- 🛠️ Tools We Tested
- ❌ AI Test Myths
- 🧠 Final Thoughts
What Is an AI Test?
An AI test is not just automation—it’s intelligent automation. Unlike traditional software testing that relies on scripts and manual oversight, AI testing applies machine learning to evaluate, adapt, and optimize systems autonomously. This includes validating data models, monitoring algorithm performance, and simulating real-world interactions.
Steps to Perform test:
- Define specific testing goals aligned with business needs.
- Choose AI-driven tools that support data modeling or task simulation.
- Execute tests and analyze outputs for accuracy and bias.
- Continuously refine models using feedback loops.
The difference lies in precision. A well-implemented AI test enhances efficiency while reducing errors that typical systems might miss.
AI Testing vs. Traditional Testing
Traditional testing is linear—run a test, record a result, adjust manually. AI testing, however, evolves with data. It can uncover blind spots, detect anomalies, and optimize itself over time.
Traditional Testing | AI Testing |
---|---|
Manual scripts | Adaptive learning algorithms |
Static datasets | Real-time input analysis |
Reactive problem-solving | Predictive and proactive adjustments |
This adaptability is why AI for business is no longer a luxury—it’s a strategic edge.
Why AI Testing Tools Matter
AI is only as strong as its foundation. An artificial AI model that hasn’t been thoroughly tested can produce biased or incorrect results, harming both users and brand reputation. With the right tools, companies can ensure transparency, safety, and scalability.
Benefits of AI Testing Tools:
- Automate time-consuming manual checks
- Detect hidden biases in datasets
- Improve model accuracy over time
- Deliver faster release cycles and reduced QA costs
For any AI development company, robust AI testing is a cornerstone of reliability.
Best Use Cases for AI Testing
AI testing isn’t hypothetical—it’s already improving outcomes in:
- Healthcare: Validating diagnostic algorithms
- Finance: Testing fraud detection models
- Retail: Optimizing recommendation engines
- Logistics: Fine-tuning supply chain predictions
Real-World Testing Strategy:
- Define your AI use case.
- Simulate real conditions using historic and live data.
- Validate outputs for bias, performance, and relevance.
- Integrate learnings into the next model iteration.
If your company uses or builds AI, testing isn’t optional—it’s essential.
Learn how to get started with ChatGPT, write better prompts, and unlock its full potential — even if you’re a total beginner.
How to Use ChatGPT
Top AI Automation Tools We Tested
Choosing the right testing tool depends on your use case. Here are three top-rated AI testing tools leading in 2025:
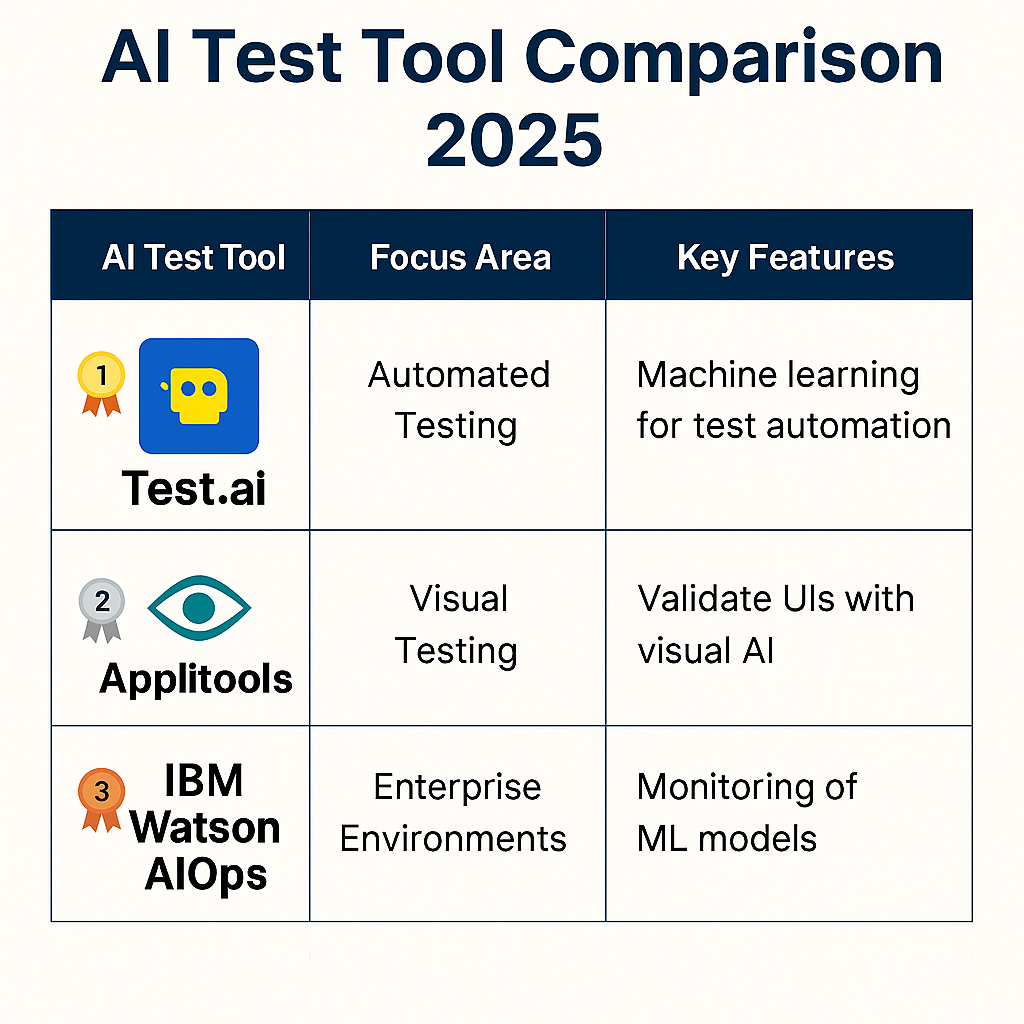
- Test.ai
Automates repetitive testing with machine learning—ideal for mobile and web apps. - Applitools
Specializes in visual AI testing, perfect for validating complex UIs with visual recognition. - IBM Watson AIOps
Enterprise-grade monitoring of ML models, ideal for large-scale infrastructure and AI for business deployment.
How to Pick the Right Tool:
- Match tool capabilities to your testing goals.
- Prepare a clean training/validation dataset.
- Test small, scale fast, and track results continuously.
These tools give any AI development company the power to scale reliably and efficiently.
Debunking Common AI Test Myths
Myth: AI doesn’t need testing—it learns on its own.
Reality: Even artificial AI models can behave unpredictably without testing, leading to bias or breakdowns in production.
Myth: AI testers replace QA teams.
Reality: AI supports testers by handling volume and complexity—human oversight is still vital for strategy and ethics.
Myth: Any automation tool = AI testing.
Reality: Only AI-powered tools with adaptive learning and prediction qualify as true AI testing platforms.
Strategic Tip:
Blend AI tools with human expertise to build systems that are not only smart—but also fair and safe.
Final Thoughts: Which AI Test Tool Is Right for You?
AI testing is no longer optional. It’s the foundation for trustworthy, scalable AI systems. Whether you’re experimenting with AI for business or building solutions as an AI development company, the right strategy protects your investment and reputation.
Done right, AI testing empowers innovation with accountability. The future isn’t just smart—it’s tested, trusted, and built to last.